It is important to use the concept of sentiment analysis while trading AI stocks, specifically for penny stocks and copyright markets where sentiment is a major factor. Here are ten top suggestions on how to utilize sentiment analysis in these markets.
1. Sentiment Analysis What do you need to be aware of
Tips: Keep in mind that prices' movements over the short term are influenced by sentiment, especially with regard to speculative stock and copyright markets.
Why: The public's sentiment is usually a key indicator of price movement and is therefore a reliable signal to trade.
2. Make use of AI to analyze a variety of Data Sources
Tip: Incorporate diverse data sources, including:
News headlines
Social media sites, like Twitter, Reddit and Telegram
Blogs and forums
Press releases and earnings announcements
Why is that broad coverage provides an overall picture of sentiment.
3. Monitor Social Media in real Time
Tips: Use AI tools such as StockTwits, Sentiment.io, or LunarCrush to monitor discussions that are trending.
For copyright: Concentrate on influencers and also discussions around particular tokens.
For Penny Stocks: Monitor niche forums like r/pennystocks.
The reason Real-time Tracking is a great tool to make the most of emerging trends
4. Pay attention to Sentiment Information
Think about metrics like:
Sentiment Score: Aggregates positive vs. negative mentions.
Buzz around the Volume of Mentions, hype or excitement around an asset.
Emotional Analysis: Measures excitement, fear, and uncertainty.
Why? These numbers can offer valuable insights into the market's psychology.
5. Detect Market Turning Points
Utilize sentiment data to find extremes of both positive and negative sentiment (market tops and bottoms).
The reason why contrarian strategies are usually efficient at extremes of emotion.
6. Combine Sentiment with Technical Indicators
TIP: Confirm sentiment using traditional indicators, like RSI, MACD or Bollinger Bands.
What's the reason? A simple emotional reaction could be misleading, whereas a scientific analysis provides some context.
7. Integration of Sentiment Data with Automated Systems
Tip Use AI trading bots with sentiment scores integrated into their decision-making algorithms.
Automated response to volatile markets allow for rapid sentiment changes to be recognized.
8. Account to Manage Sentiment
Watch out for pump-and dump schemes and fake news, in particular penny stocks and copyright.
How to use AI software to identify anomalies.
What: By recognizing manipulation it is possible to avoid fake signals.
9. Backtesting Sentiments-Based Strategies using Backtest Strategies
TIP: Take a look at how sentiment-driven trades perform under past market conditions.
Why: It ensures that your trading strategy is based upon a sentiment-based analysis.
10. Monitor Sentiments from Key Influencers
Tip: Make use of AI to identify market influencers, like prominent traders, analysts, or copyright developers.
For copyright For copyright: Focus on tweets, posts and other posts by Elon Musk (or other blockchain pioneers).
Watch for comments from activists and analysts about penny stocks.
How do they influence market sentiment.
Bonus: Combine sentiment data with basic data and data from on-chain
Tips: Combine sentiment with fundamentals (like earnings reports) for penny stocks as well as on-chain information (like the movements of wallets) for copyright.
Why: Combining various kinds of data can give an overall picture and decrease dependence solely on sentiment.
Use these suggestions to leverage sentiment analysis effectively in your AI strategies for penny stocks as well as cryptocurrencies. See the top rated ai copyright prediction for blog info including ai stock picker, ai stock trading bot free, ai trade, incite, best stocks to buy now, ai stock trading bot free, ai stock picker, ai penny stocks, stock market ai, ai for trading and more.
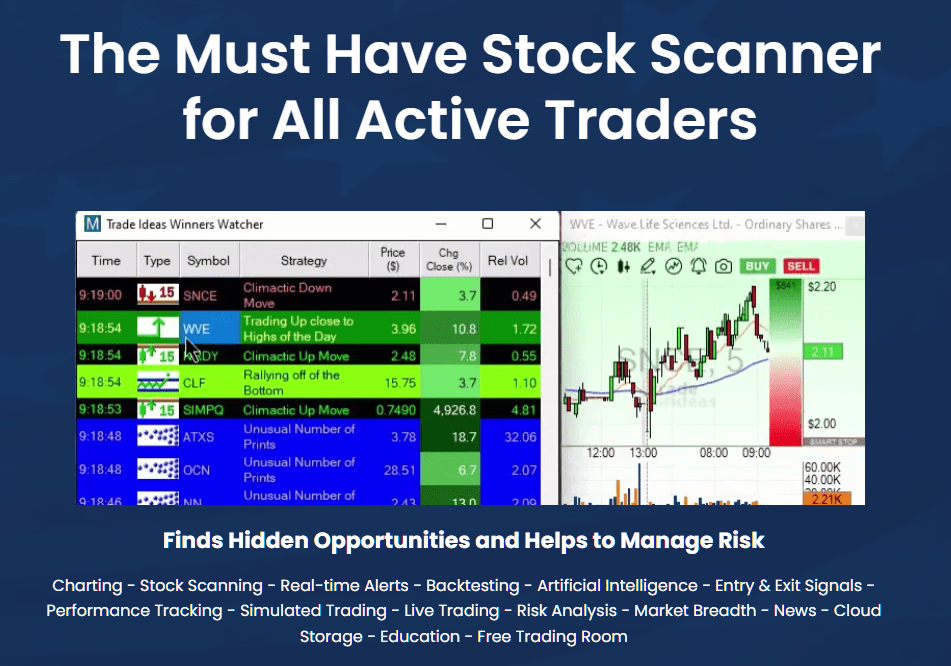
Top 10 Tips To Profiting From Ai Stock Pickers, Predictions, And Investments
It is essential to employ backtesting in a way that allows you to improve AI stock pickers, as well as improve investment strategies and predictions. Backtesting can provide insight into the effectiveness of an AI-driven strategy in the past in relation to market conditions. Here are the top 10 tips to backtesting AI tools for stock-pickers.
1. Utilize High-Quality Historical Data
Tip. Be sure that you are using accurate and complete historical data, including volume of trading, prices for stocks and earnings reports, dividends, or other financial indicators.
Why? High-quality data will guarantee that the backtest results reflect actual market conditions. Incomplete or inaccurate data can cause backtest results to be misleading, which will impact the accuracy of your plan.
2. Add on Realistic Trading and slippage costs
Tips: Simulate real-world trading costs, such as commissions and transaction fees, slippage and market impact during the process of backtesting.
The reason: Not accounting for slippage and trading costs can overstate the potential returns of your AI model. These variables will ensure that your backtest results closely match the real-world trading scenario.
3. Test different market conditions
Tips - Test the AI Stock Picker for multiple market conditions. These include bull markets and bear markets, as well as times with high volatility (e.g. markets corrections, financial crises).
Why: AI algorithms can be different under different market conditions. Testing under various conditions can make sure that your strategy can be able to adapt and perform well in various market cycles.
4. Test with Walk-Forward
TIP : Walk-forward testing involves testing a model by using a rolling window historical data. Then, test its results using data that is not included in the test.
Why: Walk-forward tests help assess the predictive powers of AI models based upon untested evidence. This is a more accurate gauge of real world performance than static backtesting.
5. Ensure Proper Overfitting Prevention
Beware of overfitting the model through testing it using different time periods. Also, make sure the model isn't able to detect irregularities or create noise from previous data.
What happens is that when the model is tailored too closely to historical data, it is less reliable in forecasting future trends of the market. A model that is balanced will be able to adapt to different market conditions.
6. Optimize Parameters During Backtesting
TIP: Backtesting is excellent method to improve important variables, such as moving averages, positions sizes, and stop-loss limits, by adjusting these variables repeatedly and evaluating the impact on return.
Why optimizing these parameters could increase the AI model's performance. However, it's important to ensure that the optimization does not lead to overfitting, as previously mentioned.
7. Drawdown Analysis and Risk Management Integration of Both
TIP: Include risk management techniques such as stop losses as well as ratios of risk to reward, and the size of your position during backtesting. This will enable you to determine the effectiveness of your strategy when faced with large drawdowns.
Why: Effective risk-management is critical for long-term profit. It is possible to identify weaknesses by analyzing how your AI model handles risk. After that, you can modify your strategy to get better risk-adjusted return.
8. Examine key metrics that go beyond returns
Tip: Focus on key performance indicators beyond the simple return like Sharpe ratio, maximum drawdown, win/loss, and volatility.
The reason: These metrics give you a more comprehensive knowledge of your AI strategy's risk-adjusted returns. If you solely focus on the returns, you might overlook periods with high risk or volatility.
9. Simulate a variety of asset classes and strategies
Tip: Test the AI model with different types of assets (e.g. stocks, ETFs and copyright) as well as various investment strategies (e.g. mean-reversion, momentum or value investing).
The reason: Diversifying backtests across different asset classes lets you to assess the adaptability of your AI model. This ensures that it will be able to function in a variety of markets and investment styles. This also makes the AI model to work when it comes to high-risk investments such as cryptocurrencies.
10. Make sure you regularly update and improve your backtesting approach
Tip: Continuously refresh your backtesting framework with the latest market data and ensure that it is constantly evolving to reflect the changing market conditions and brand new AI models.
Why: Markets are dynamic and your backtesting needs to be too. Regular updates ensure that your AI models and backtests remain effective, regardless of new market trends or data.
Use Monte Carlo simulations to assess the level of risk
Use Monte Carlo to simulate a number of different outcomes. This can be done by performing multiple simulations using different input scenarios.
The reason: Monte Carlo models help to better understand the potential risk of various outcomes.
Follow these tips to evaluate and optimize the performance of your AI Stock Picker. If you backtest your AI investment strategies, you can make sure they are reliable, robust and able to change. Take a look at the top ai for stock market for site recommendations including best stocks to buy now, best ai stocks, ai stocks to buy, ai trading app, ai penny stocks, incite, ai trading software, ai copyright prediction, best ai stocks, ai trading and more.
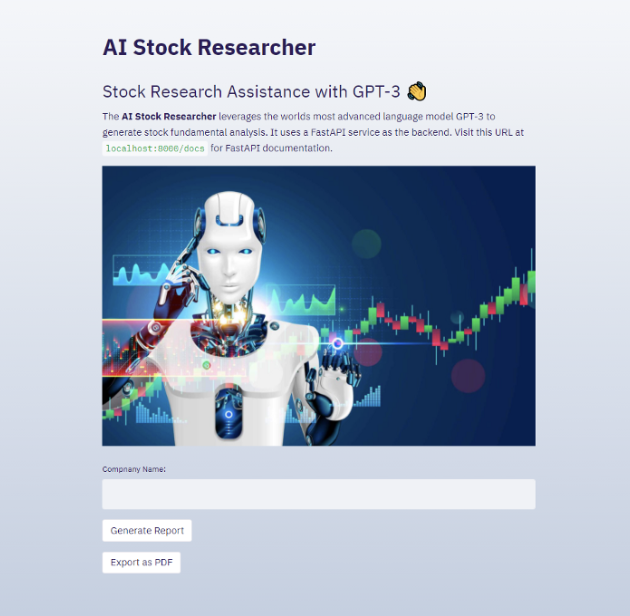