The importance of focusing on risk is essential for AI trading in stocks to be successful, especially in high-risk markets. Here are 10 top strategies to help you implement risk management strategies into your AI trading.
1. Define Risk Tolerance
Tips: Determine the maximum amount of loss that will be accepted for every trade, drawdowns on a daily basis and portfolio losses.
Why: Understanding your risk threshold helps you set precise parameters for your AI trading system.
2. Automated Stop Loss and Take-Profit orders
Tips: Make use of AI technology to automatically adjust the amount of take-profit or stop-loss based upon volatility and market conditions.
What's the reason? Automated safeguards minimize potential losses and lock in profits without emotional interference.
3. Diversify Your Portfolio
Tips: Spread the investments across a variety of sectors, assets and markets (e.g., mix penny stocks, stocks with a large capital, and copyright).
The reason is that diversification can reduce exposure to the risks of one asset in addition to balancing the potential for profits and losses.
4. Set Position Sizing Rules
Make use of AI to calculate the dimensions of your position Based on:
Portfolio size.
Risk per transaction (e.g. 1 - 2% of the total value of portfolio).
Asset volatility.
Size of the position is essential to ensure that you do not overexpose yourself in high-risk trading.
5. Monitor volatility and adjust your strategies
Tip: Monitor market volatility by using indicators such as the VIX (stocks), on-chain data, or any other indicators.
Why is higher volatility a call for tighter risk control, more adaptive trading strategies, and more trading levels.
6. Backtest Risk Management Rules
Include risk management factors such as size of the position and stop-loss in backtests to evaluate.
The reason: Testing your risk measures ensures that they're a viable option under a variety of market conditions.
7. Implement Risk-Reward Ratios
Tip. Make sure every trade you make has the right risk-reward ratio, for example 1:3 (1:3 = $1 at risk x $3 gain).
The reason is that consistent use of favorable ratios improves long-term profitability even in the event of occasional losses.
8. AI that detects and responds to any anomalies
Tips: Set up algorithms for detecting anomalies to spot unusual trading patterns like sudden increases in volume or price.
The reason is that early detection allows you to modify your strategies or stop trading prior to the onset of a major market movement.
9. Hedging Strategies to Incorporate
Hedging techniques such as options or futures can be used to limit risk.
Penny Stocks hedge with sector ETFs or other assets.
Use stablecoins to protect your investment portfolio or inverted exchange-traded funds.
The reason: Hedging helps protect against adverse price movements.
10. Continuously monitor and modify Risk Parameters
Tips: Re-evaluate and revise the settings of your AI trading system's risk settings as market conditions evolve.
Why: Dynamic risk-management ensures that your plan is relevant across different market conditions.
Bonus: Use Risk Assessment Metrics
Tip: Evaluate your strategy using metrics like:
Max Drawdown Maximum Portfolio Fall from peak to trough.
Sharpe Ratio: Risk-adjusted return.
Win-Loss: Ratio between the amount of profitable trades to the losses.
What are they? They offer insight into the performance of your strategy and risk exposure.
If you follow these guidelines to implement these tips, you can develop a robust risk management framework which improves the efficiency and safety of your AI trading strategies for penny stocks and copyright markets. Have a look at the top stock trading ai blog for website advice including ai predictor, investment ai, ai trading software, ai stock price prediction, penny ai stocks, ai stock picker, incite, best ai penny stocks, ai predictor, best ai trading bot and more.
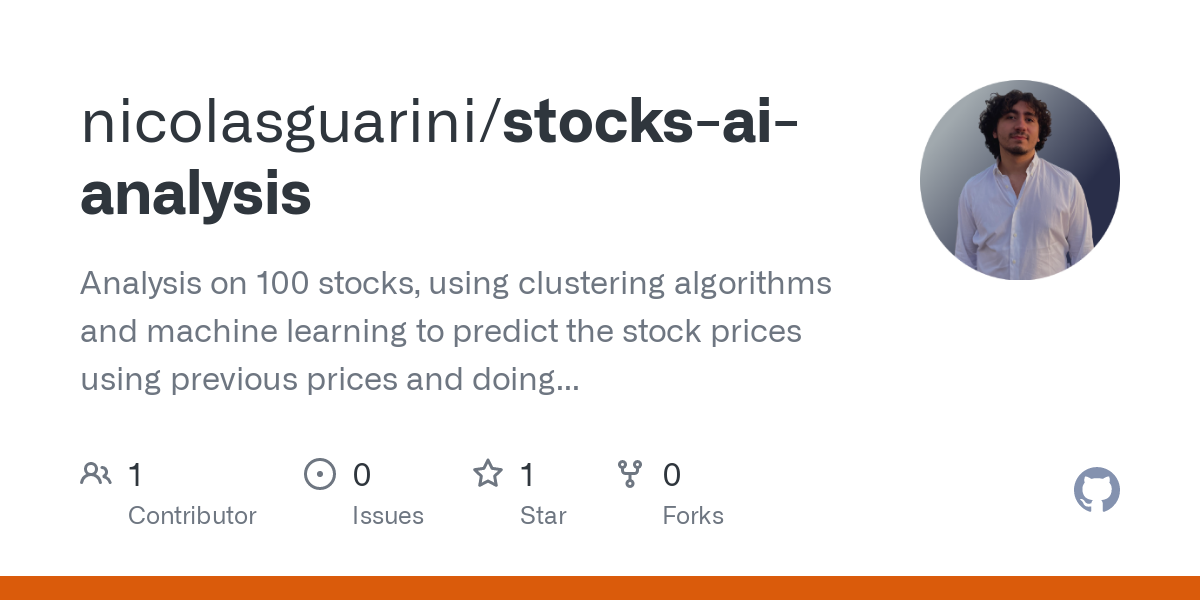
Top 10 Strategies To Use Ai Stock-Pickers To Improve The Quality Of Their Data
AI-driven investment predictions, AI-driven forecasts and stock selection are all dependent on the quality of data. AI models can provide more accurate and reliable predictions if the data is of high-quality. Here are ten top guidelines for ensuring quality data in AI stock analysts:
1. Prioritize data that is well-structured and clear
TIP: Ensure your data is free from mistakes and is organized in a consistent way. This includes removing double entries, addressing the absence of values, and ensuring the integrity of your data, etc.
Why is that clean and organized information allows AI models process information more effectively. This results in more accurate predictions and less mistakes made in decisions.
2. Timeliness of data and real-time data are essential
TIP: To predict future events using real-time information, including the price of stock and trading volume, earnings reports and news sentiment.
Why: The regular updating of data assures that AI models are correct especially when markets are volatile, such as copyright or penny stocks.
3. Data from trusted providers
TIP: Choose the data providers that are reliable and have gone through a thorough vetting process. This includes financial statements, economic reports as well as price feeds.
The reason: Using reliable data sources reduces the risk of errors and inconsistencies in data, which could affect AI model performance or lead to incorrect predictions.
4. Integrate data from multiple sources
Tips. Combine different data sources including financial statements (e.g. moving averages) news sentiment, social data, macroeconomic indicators, and technical indicators.
The reason is that a multi-source approach can provide a more comprehensive view of the market, allowing AI to make more informed decisions by recording various aspects of stock behavior.
5. Backtesting historical data is the focus
Tip: Collect high-quality historical data for backtesting AI models to test their performance in different market conditions.
The reason is that historical data can help you refine AI models. You are able to test trading strategies by simulation, to determine potential returns and risks as well as ensure AI predictions that are robust.
6. Verify the Quality of data continuously
Tip: Regularly audit data quality and look for any inconsistencies. Update information that is outdated and make sure the information is accurate.
What is the reason: Consistent validation assures that the data you input into AI models is reliable, reducing the risk of making incorrect predictions based upon inaccurate or obsolete data.
7. Ensure Proper Data Granularity
Tip - Choose the level of granularity you think is best to your strategy. For example, you can, use regular data or minute-by-minute information when you are investing long-term.
Why? The right level of granularity for your model is critical. For example, short-term trading strategies benefit from high-frequency data while investing for the long term requires more detailed, low-frequency data.
8. Incorporate other data sources
Utilize alternative sources of data, such as satellite imagery or sentiment on social media. You can also scrape the internet to discover the latest trends in the market.
What's the reason? Alternative data provides unique insight into market behavior, giving your AI system a competitive advantage by identifying patterns that traditional sources of data might miss.
9. Use Quality-Control Techniques for Data Preprocessing
Tip: Implement quality control measures such as normalization of data, detection of outliers and feature scaling in order to process raw data prior to entering it into AI models.
Why is it important to preprocess data? It ensures that the AI model is able to interpret the data with accuracy. This reduces the chance of errors in predictions and enhances the overall performance of the AI model.
10. Monitor Data Drift and Adapt Models
Tips: Always monitor data drift (where the characteristics of the data change with time) and modify your AI model accordingly.
Why: Data drift can impact the accuracy of your model. By recognizing, and adapting, to changes in patterns in data, you will ensure your AI is effective over time, particularly on dynamic markets such as cryptocurrencies or penny stocks.
Bonus: Maintaining the Feedback Loop to ensure Data Improvement
Tip: Set up a feedback loop that ensures that AI models continuously learn from the new data. This can help improve the data collection and processing method.
Why: By using feedback loops that improves the quality of data and adjust AI models to current market conditions.
Emphasizing data quality is crucial for maximizing the potential of AI stock pickers. AI models are able to make more accurate predictions when they are able to access high-quality data that is current and clean. This allows them to make better investment choices. By following these guidelines, you can make sure that you've got the most reliable information base to allow your AI system to predict and make investments in stocks. Follow the recommended copyright predictions tips for site advice including best copyright prediction site, ai stock trading app, ai trading software, ai for stock market, best ai stock trading bot free, ai investment platform, ai copyright trading bot, ai stock price prediction, incite, best ai stocks and more.
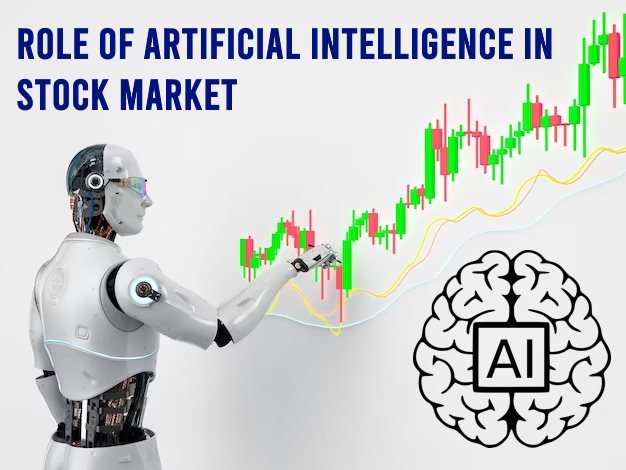